Overcoming Clinical Data Aggregation Challenges
By Ben McGraw. Strategic Marketing, Revvity Signals Software
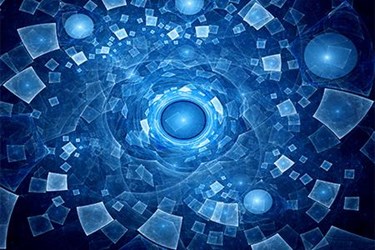
access the White Paper!
Log In
Get unlimited access to:
Trend and Thought Leadership Articles
Case Studies & White Papers
Extensive Product Database
Members-Only Premium Content
Welcome Back! Please Log In to Continue.
X
Enter your credentials below to log in. Not yet a member of ECM Connection? Subscribe today.
Subscribe to ECM Connection
X
Subscribe to ECM Connection